Everything you always wanted to know about AI, but were afraid to ask.
This article was originally published in 2017, but we think it's freshly relevant today.
AI is hot. But, what, exactly is it and what can it really do? Last week at the ABA Wealth Management and Trust Conference, Mark Nitzberg and Bill Martin sat down to speak as part of a presentation on the “Transformation of Advice and Work in a World of Thinking Machines.”
We know Mark as Smartleaf's co-founder and current senior advisor. Mark holds a PhD in Computer Science from Harvard University and is currently the Executive Director of the University of California Berkeley Center for Human Compatible Artificial Intelligence (EDUCBCHCAI for short). Mark recently published Solomon's Code: Humanity in a World of Thinking Machines. Bill Martin is Intrust Bank’s CIO (as well as a long-time Smartleaf user). Last year, Bill published The Smart Financial Advisor: How financial advisors can thrive by embracing fintech and goals-based investing. Their conclusion: AI will not put advisors out of business. It will free advisors from doing rote tasks, enabling them to do what only humans can do-build meaningful relationships of trust and advice.
Last year, we sat down with Mark to ask him about AI (Artificial Intelligence) and here's what we learned.
We’re hearing a lot about AI. Let’s start at the beginning. What is AI?
AI describes systems that perform tasks we normally associate with human cognition. In the science fiction and Hollywood versions, AI achieves or exceeds human-level intelligence. This (currently) fictional type of AI is called "Strong AI" or AGI for Artificial General Intelligence. Talking to Siri or Alexa might give the impression that we are getting close to creating AGI, but most computer scientists think it is decades away (at least). But while AGI may be far away, computer scientists are taking the potential dangers of AGI seriously. SkyNet (from the movie Terminator) trying to destroy humanity is Hollywood’s version of the worst-case scenario, but it’s not the one that is most worrisome. You run into real issues even with self-driving cars (would making passenger safety paramount lead to the car running over a dozen pedestrians rather than swerving?). Thinking early about how to make safe AI—AI whose objectives are aligned with human values—is the focus of CHAI, the Center for Human-Compatible AI, where I work.
More colloquially, AI is used to describe any complex software that does something that a human does, possibly better. In this sense, Smartleaf’s rebalancing analytics are AI—they can rebalance complex portfolios, probably better than most humans. Incidentally, Smartleaf’s chief architect, Robert Thau, has a PhD in Computational Neuroscience from MIT.
But all the hoopla you’re hearing about AI these days is largely about neural networks and deep learning.
What are neural networks and what is deep learning?
It’s a way to train computers using vast data sets of test cases where the right answer is known, in the hope that you’ll get it right when the answer isn’t known. For example, if you wanted to train a neural network to recognize kittens, you’d show it lots (perhaps millions) of photos of kittens, as well as lots of photos that aren’t of kittens. With every photo, the system guesses, and there are tweaks to internal “paths” based on right and wrong answers. Speech recognition, face recognition, and translation are all examples of this type of AI. One of my favorite applications of deep learning is colorization of black and white films. You train a network using real color films that are de-colored, frame by frame. The network learns what patterns should be colored in what shades based on the patterns within the billions of training frames. Then you apply the network to a black and white film that has never had color. The results are striking.
Why is it hot now?
AI is hot now because about seven years ago, we had some real breakthroughs. The first breakthrough came in the form of a leap in accuracy of systems recognizing photos of objects (like, well, kittens). This set off a series of leaps in speech recognition, language translation, and other applications.
Though these first really impressive successes were recent, neural networks have been around for decades. They were really hot within computer science in the 1970s, but not much came of it. There were all these embarrassing failures, and most computer scientists dismissed it as a dead end. Famously, the army tried to train a computer to recognize tanks, but all the training photos of tanks were taken on a cloudy day, so the computer just learned to recognize clouds.
However, it turns out computer scientists gave up too early. The methodology was right—it just needed faster computers and lots more data to work.
What are the types of problems that are solvable by AI?
You need to be able to train AI systems with lots of data, and either known correct answers for the data, or a way to tell that the output is good. This is called a reward function (or, in the negative, a loss function). You may have noticed that the “captcha” challenge questions you see on websites to screen out bots where you have to identify something often come in pairs. The second entry is not for screening. It’s so you can help provide a set of answers to train some AI application.
Can you give examples?
That’s easy! Deep learning powers Google Translate’s translation, Alexa's, Siri's, and Google Home’s speech recognition; IBM Watson’s cancer diagnosis (more accurate than doctors, at least measured on a certain set of images); Facebook’s—and pretty much every other company’s—face recognition and picture tagging; and dozens of other systems with great results. You’ve probably read about Google Deep Mind’s “go” program beating the world champion. But that’s a parlor trick compared to some of these life-changing systems.
Ok. Let’s turn to financial services applications. What’s out there?
Well, the obvious one is using AI to pick securities to buy and sell. You’ve got lots and lots of data—second-by-second real-time price history on thousands of securities.
Would it work if I just threw every security trade tick and every piece of data on the internet—weather in Brazil, cat videos and Little League scores—into a neural net? Would I get a useful stock price predictor?
Not likely. It would find patterns, but they aren’t likely to be causal. You’d probably just get a real-world illustration of the difference between correlation and causation. If you narrowed down, you might end up with something intuitive, like a connection between weather patterns in key agricultural regions and the price of restaurant stocks.
What are some other fintech applications? What about financial planning?
Financial planning seems like it’s ripe for improvement, but it’s not clear that AI is currently able to help. The problem goes back to having lots of data with known answers for the reward function. What is the “correct answer” with financial planning? You might come up with something, but the challenge lies in defining a good outcome. Analyzing investment behavior to identify what inputs generate the more desirable outcomes would be a valuable social study, a study for which few organizations have access to the necessary data.
So what do you think will be the first big applications in wealth management?
My best guess would be sales. Firms like Salesforce and other CRM vendors are working on using AI to do a better job of identifying prospects and guiding the sales process (“you should follow up with a letter”). It can also guide advisors to clients who may be at risk of leaving or who are good candidates for an expanded relationship. Salesforce may be in a position to dominate this if they can induce its large installed client base to share its data.
Last question: if you were to get tired of answering these Q&As, would it be possible to create an AI program (the Nitzbot3000?) that answered them for you?
As a matter fact, this isn’t all that far-fetched. You’d combine two AI projects. The first would be to understand the questions and come up with reasonable replies. This is what Siri does. You’d train the system on lots of questions with known answers. The next step would be to train the system to respond in a manner that sounds like me. To get there, you’d take everything I’ve ever written to get a probabilistic sense of word choice and order. There’s a company (Replika) that’s trying to do this, though they still have a long way to go from a superficial appearance of a good answer to actually capturing the tone, narrative arc and other subtleties needed to hold a decent conversation. But I think it’s possible. I, for one, look forward to meeting me.
For more on this topic, check out Automated Rebalancing & Specialization.
![]() |
Mark Nitzberg Executive Director of the |
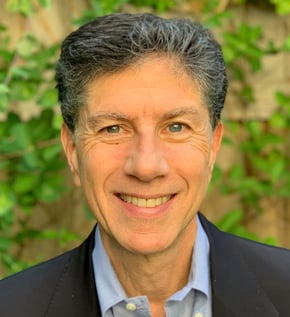
COMMENTS